

This means that the Solver works with a group of cells called decision variables that are used in computing the formulas in the objective and constraint cells. In Excel, you can use Solver to find an optimal value (maximum or minimum, or a certain value) for a formula in one cell called the objective cell, subject to certain constraints or limits, on the values of other formula cells on the worksheet. Then, one or more other variables are changed repeatedly, subject to the specified constraints, until you discover the best values for the target variables. Instead of setting a specific target value for a variable, the goal is to find the optimum value for one or more target variables, under certain constraints. The multiscale hybrid strategy is able to offer the best result.Solver is a Microsoft Excel add-in program you can use for optimization in what-if analysis.Īccording to O'Brien and Marakas, optimization analysis is a more complex extension of goal-seeking analysis.

With a proper initial guess and a set of effective weight coefficients, MOAdjoint can most efficiently solve large-scale continuous problems, particularly if model uncertainty is considered.
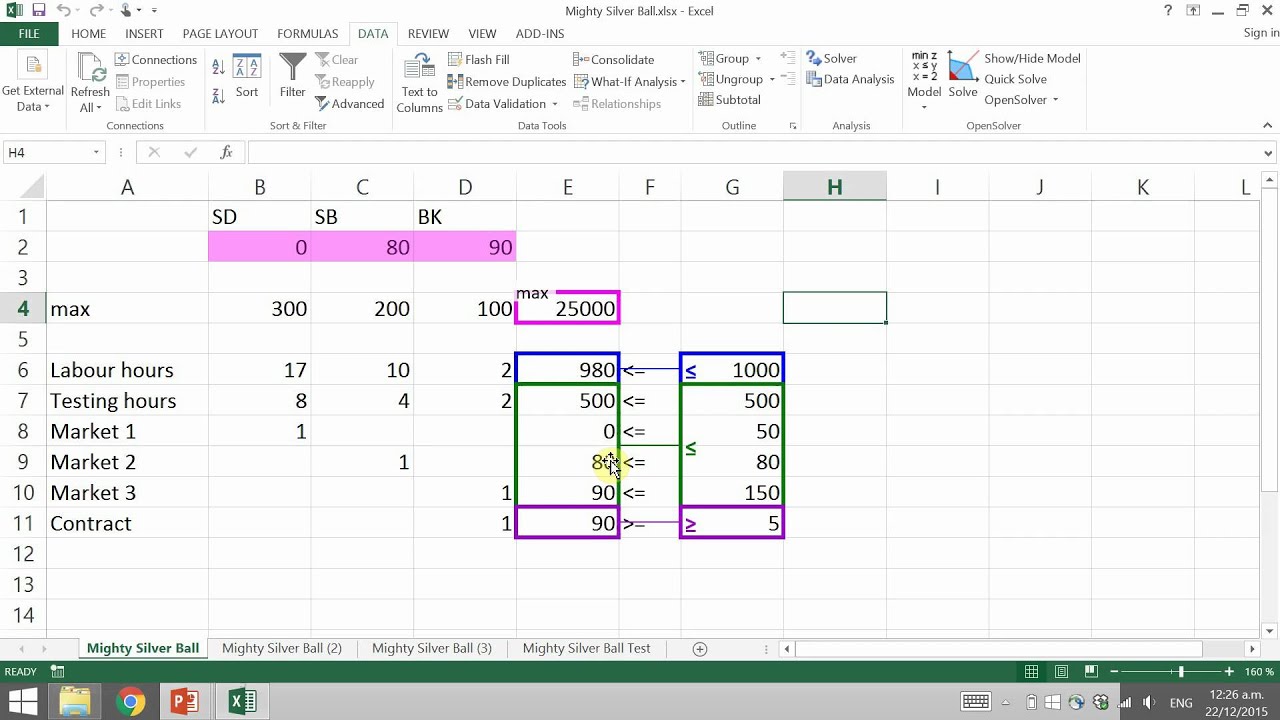
MOPSO seems particularly suitable for medium-scale RM problems, mainly because of its relatively fast convergence speed and efficient recovery of the Pareto front. Results show that all three methods can yield improved solutions.
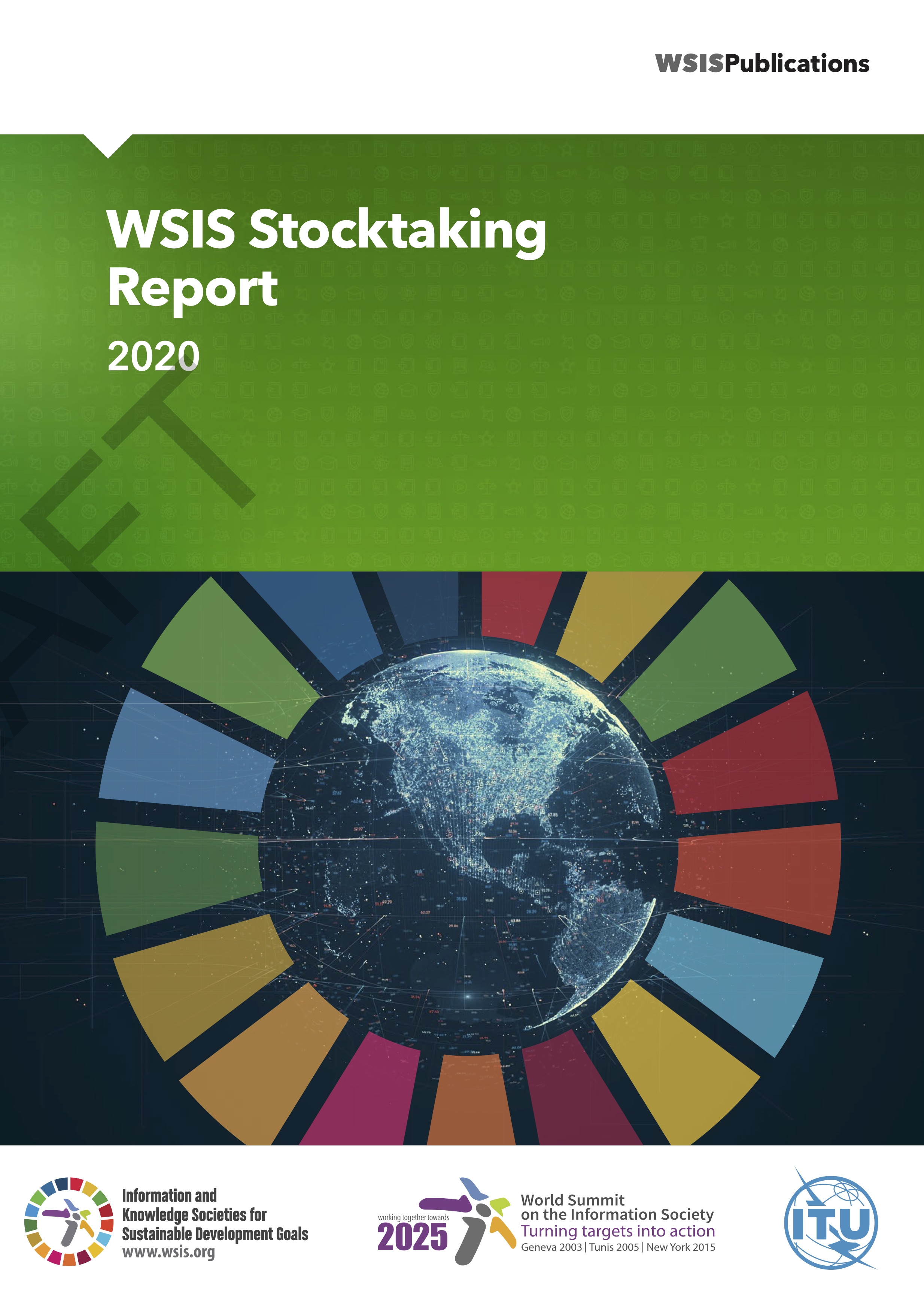
An example is used to compare these methods. To take advantage of the strengths and overcome the drawbacks of these methods, a multiscale hybrid strategy is further formulated for solving complex, large-scale optimization problems by combining these methods at various scales. Their advantages and disadvantages are highlighted. MOPSO is another population-based method, which combines a Pareto technique with particle-swarm optimization (PSO) for a wide spectrum of optimization problems. MOGA is a population-based method, which combines a Pareto-ranking technique with genetic algorithm (GA) to address small-scale (discrete) problems. An adjoint method is used to efficiently compute the derivatives of objective functions with respect to decision variables, and a sequential quadratic-programming method is used for optimization search. MOAdjoint combines a weighted-sum technique with a gradient-based method for solving large-scale continuous problems that have thousands of variables. Considering the complexity and diversity of MOO problems in model-based RM, we develop three MOO methods-MOAdjoint, MOGA, and MOPSO-in this work to address various oilfield-development problems. Multiobjective optimization (MOO), which accounts for several distinct, possibly conflicting, objectives, is expected to be capable of providing improved reservoir-management (RM) solutions for efficient oilfield development because of the overall optimization of subsurface flow.
